How text analytics delivers customer experience value
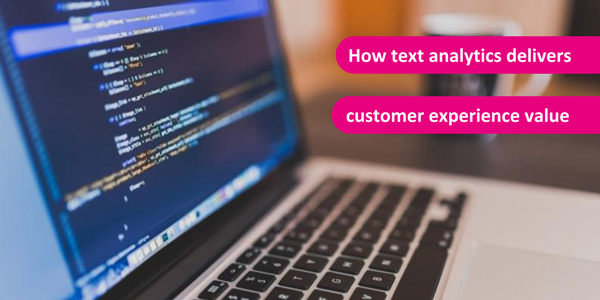
Artificial intelligence (AI) has the power to transform customer experience, enabling brands to meet rising consumer expectations through tailored, high quality, relevant service that drives increased loyalty.
In my previous series of blogs I explained the basic AI terms, including bots and chatbots, and the impact they have on CX, and now I want to turn my attention to text analytics. This is at the heart of successful AI, with Natural Language Processing (NLP) used to extract meaning from written messages, such as emails, Tweets or chat sessions. How does it work and how do you benefit from it?
Let’s start by assuming we’re sitting in a company and a consumer decides to make contact by sending a message through a digital channel. In this case we’ll say it is an email, although it could equally be a tweet, Facebook message or a chat session. Here are the eight major steps that AI software such as Eptica’s goes through in order to understand and extract real meaning from the message:
- Language detection. The first step is to understand what language is actually being used in the message, including whether it is a particular variant, such as American English. This enables the correct analysis to be performed and the right understanding and terms used.
- Cleaning. This gets rid of any sentences or words without any meaning, such as “Dear XYZ” and “Thanks”, removing any noise that is not related to the message, and thus leaving the real content ready to be analyzed.
- Syntax analysis. What is the structure of each sentence in the message? For example, which word is the subject, which is the verb?
- Normalization. This involves ensuring that similar terms are brought together. For example, “buying” and “bought” are both variants of the verb “buy”, so should be seen as essentially having the same meaning. Normalization reduces the complexity of text analytics as common terms are identified early.
- Contraction resolution. Often people abbreviate words (such as “did not” to “didn’t”), and this needs to be expanded for clarity.
- Clause level detection. When you break down individual sentences you find that they often contain different clauses, sometimes with different meanings. For example, “I liked the food, but the waiter was rude”, has two clauses that need to be identified separately. Simply reading the first clause and ignoring the second would give a completely wrong impression of the experience the consumer has had.
- Name and entity recognition. Certain words, such as dates, places or brand names, provide important context to the conversation. Identifying these enables the text analytics engine to better understand what the message is about, helping improve the accuracy of the answer it provides.
- Semantic analysis. Once the message has gone through the steps listed above, NLP can be used to understand its meaning. As part of this it can look at the context of the conversation – for example the sentences “My laptop is small and easy to carry” and “My hotel room is small” both feature the word small, but in one it is positive and the other negative. Sentiment can also be measured, looking at the split of positive and negative terms in the message. Given that most incoming messages are around a limited number of topics, identifying the theme of the conversation (such as about extending a credit card limit), is also important, meaning that systems should be sourced with a list of relevant themes for that industry and organization.
Once the text analytics process is complete, our bots will then look through the knowledge base for the most relevant answer. This is then provided either direct to the consumer (as in the case of self-service, chatbots and automated conversations), or as a response or template that is intelligently routed to the person within the organization best equipped to reply, so that they can deliver a tailored, personalized answer, by email, chat or social media. To ensure that the system is continually improving, our bots are self-learning in two ways:
- The more a template or answer is used the more pertinent it becomes to that query, and therefore the more likely it is to be provided again if a similar question is asked.
- The bots learn through feedback from agents and consumers on the relevance of responses, enabling them to ensure they are always using the best possible answer
All of this is under the supervision of a human, to avoid any potential issues where feedback is deliberately used to lead bots astray - an issue which Microsoft came across with its Tay chatbot on Twitter.
While text analytics is not a new field, the whole science of linguistics is both extremely broad and is evolving very fast. Therefore to keep pace, and to ensure that the software that is created is relevant to the needs of customer experience, it is vital that brands choose to work with organizations that both own and develop their own text analytics and AI capabilities and have a deep understanding of the CX market and its particular requirements. That way AI will deliver real, lasting value to your CX activities.
Comments